Keep Your Data Up-to-Date
If your measurement data is weeks or months out of date, you’re flying blind—you can’t react to emerging problems and you won’t be able to.
You want to be able to track your data as it’s ingested and processed and analytics pipeline. Without good visibility, it’s easy to import the same data twice or miss a data file. Even if you’re careful, things can go wrong! That’s why we provide real-time feedback on all the data we ingest.
Read about how we process gigabytes of data every day from railroads like you
Automatically Remove or Correct Bad Data
Even the most modern measurement systems routinely report incorrect data. Perhaps weather or other conditions affected the instruments, or an instrument was miscalibrated and all its measurements are off by an inch.
We usually find that clean data is more important than obscure mathematical wizardry. Machine learning doesn’t work unless the data it’s processing is correct—otherwise “garbage in, garbage out.” Much of the academic work in this space ignores this critical prerequisite.
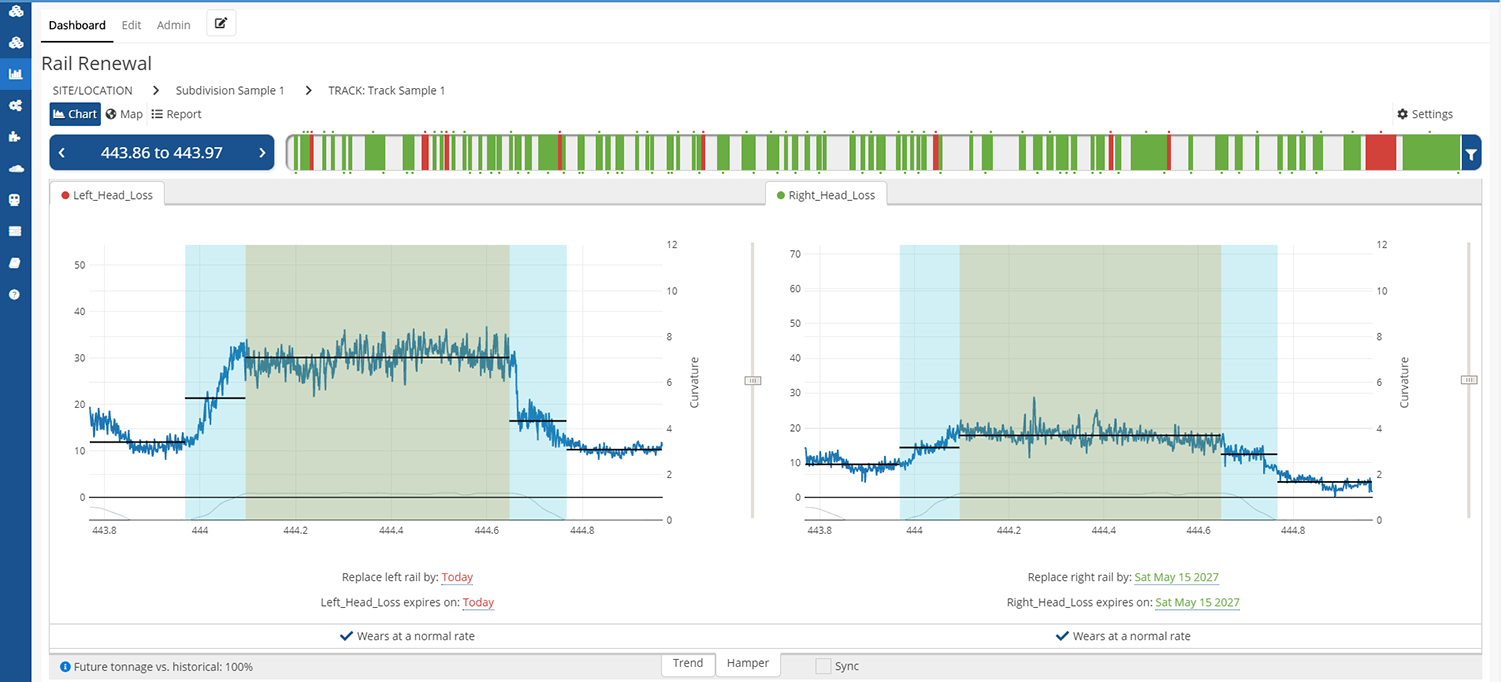
For example, rail wear data often exhibits large, meaningless spikes, or shows gaps where it couldn’t measure data. Sometimes when you look at flawed data, you can see how it should be corrected, but sometimes all you can say is that it’s wrong. In any case, there’s way too much data to try to correct manually. Thankfully, with tools from digital signal processing and using statistical analyses of the measurement and related events, we can often reconstruct data more accurately than you’d be able to do by hand!
But sometimes a measurement event is consistently wrong due to calibration errors. It’s running hot, recording biased data. While these kinds of errors tend to cancel out over time, they’re a big problem when you’re trying to understand the current state of your network—can you trust the numbers in front of you? We’ve invested heavily in understanding this problem and can often correct such errors automatically with enough data, but of course there’s no replacement for accurate measurements.
Actionable Reporting for Decision Makers
At the end of the day, your system needs a great interface so that you can plan maintenance. We believe that a) you should have high-level summaries to save you time, and b) you should still have complete visibility into the system, so you can drill down to verify anything you want.
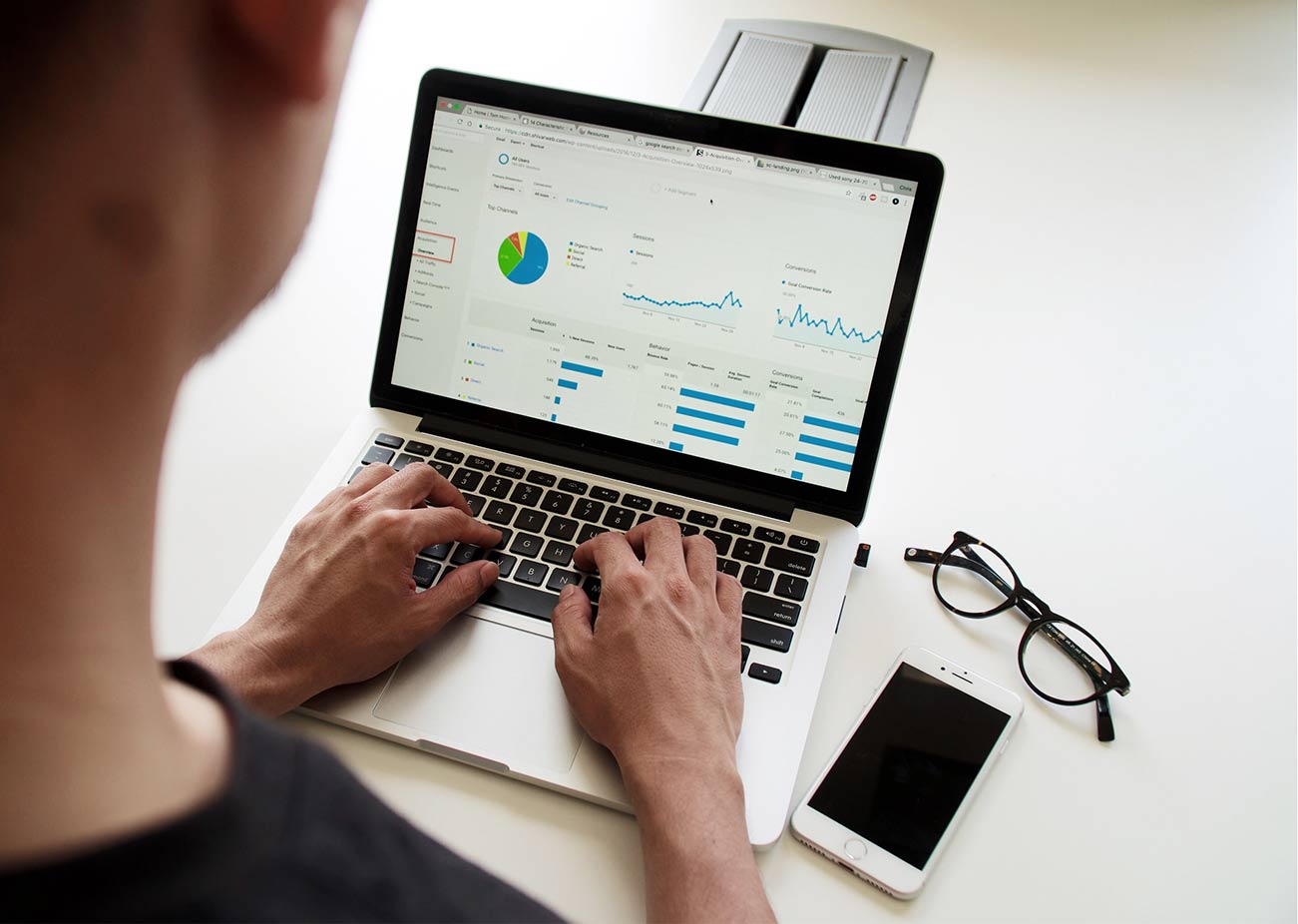
You need to rapidly review deteriorating regions. Maybe there are 10 places on the track that need attention. You shouldn’t have to look through a whole report to check those! You should be able to check the places that are projected to wear out this year, next year, and the year after that.
The system should meaningfully segment your track. Often you’ll want to replace the whole body of a curve, but sometimes just half the curve needs to be reviewed because the other half was repaired last year. At the same time, the system should sensibly merge different repair regions if they’re close together. Oh, and if part of the same track fails this year, and another part fails in two years? You want a report that thinks like you do.
You should be able to flexibly set tolerances. Regulations mean that sometimes sharp curves have different wear thresholds than slight curves. Different rail weights can have different tolerances, too. You need to be able to mix and match these rules so that the system understands the actual decision making process.
The system should be completely configurable. You’re a power user! Maybe you want to up the projected usage next year, or tweak the rules for what counts as replaceable. Or maybe you just want to adjust some filters so you can take a great screenshot. Go wild.
And . . . you should be able to easily see actual wear data if you want to in order to validate the system’s verdict. At the end of the day, summaries can be wrong. If you can see the data for yourself, and compare it with historical measurements, you don’t have to trust the computer. (But we strongly believe in giving you reasons to trust the system . . . it’s a lot faster that way.)
Scale it with the Cloud
Okay, but what about doing all of this in real-time for thousands of miles of track? It’s one thing to run a model on your laptop. But you need a production-ready system that can handle round-the-clock data, recover from errors, and let you know if there’s a problem.
We’ve seen lots of organizations try to roll their own infrastructure in this area, but it almost always ends poorly. Perhaps you planned for 100 giga-chunks of work, but your new data system is now giving you 500 giga-chunks, and your system has slowed to a crawl. Thanks to our state-of-the-art cloud integration, we can spin up hundreds or thousands of cores automatically to quickly process data in parallel.
But it’s not all challenges! There’s also lots of opportunities from comparing data across your entire network. It’s a lot easier to find a signal from the noise when you can compare all your wear data at similar locations. You can even discover facts like “this subdivision is wearing down 50% faster than a typical subdivision in our network.” And when you start asking questions armed with this kind of data, you’re truly on the path to preventative maintenance. Fix it early.